Weather-related disasters are more prevalent and catastrophic. Generation, transmission, and distribution power utilities are adopting a data-driven approach with Artificial Intelligence (AI) to improve reliability (reducing outages). They are using more technology to increase resiliency (ability to withstand longer outage events). Some utilities use historical and real-time data to predict what is going to happen, and where it will happen. Their goal is to reduce the risks when disasters strike.
Data silos restrict many utilities from using their historical data. By aggregating data sources from different systems in the PwrMetrix® platform, utilities can see actionable insights. These insights are portrayed with visual heat maps and AI predictors. It allows utilities to effectively adjust their planning and operational work plans.
What to do before the storm?
- Clean up your data with the AI error detector.
- Eliminate error codes of the unknown outages and incorrect feeder locations.
- Find best and worst substations and feeders based on multiple GIS data overlays.
- Identify and predict the most probable cause of outages, such as vegetation management issues, lighting, snow etc.
- Visualize substations and feeders affected by flood, hurricane, wildfire that increase power restoration times and increase lineman risk.
- Sectionalize areas to lower the number of meter outages.
- Determine the probability of a pole-to-pole span for at-risk areas.
- Run AI predictors to deploy crew and resources to replace the highest probable failing devices.
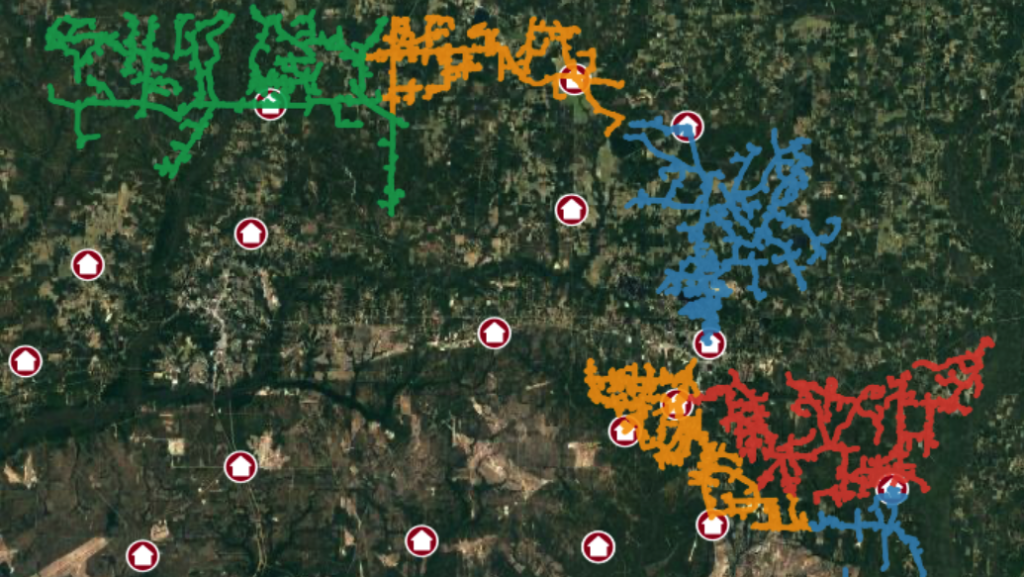
What to do during the storm?
- Pull weather trends to predict real-time risks.
- Visualize, predict, and decide to reassign the operations crew.
- Predict devices in the path of lighting or high winds trajectory.
- Create real-time reports of total customers affected by the storm.
- Focus on reducing restoration by clustering events and resources.
- Reduce the amount of waiting for replacement materials by predicting needed resources.
- Identify flooding areas to reassign response times and alert local authorities.
- Estimate time of failure to reduce equipment replacement wait times.
- Visualize and cluster related events to use lineman resources more efficiently.
- Decrease logistics issues and restoration times by positioning replacement materials closer.
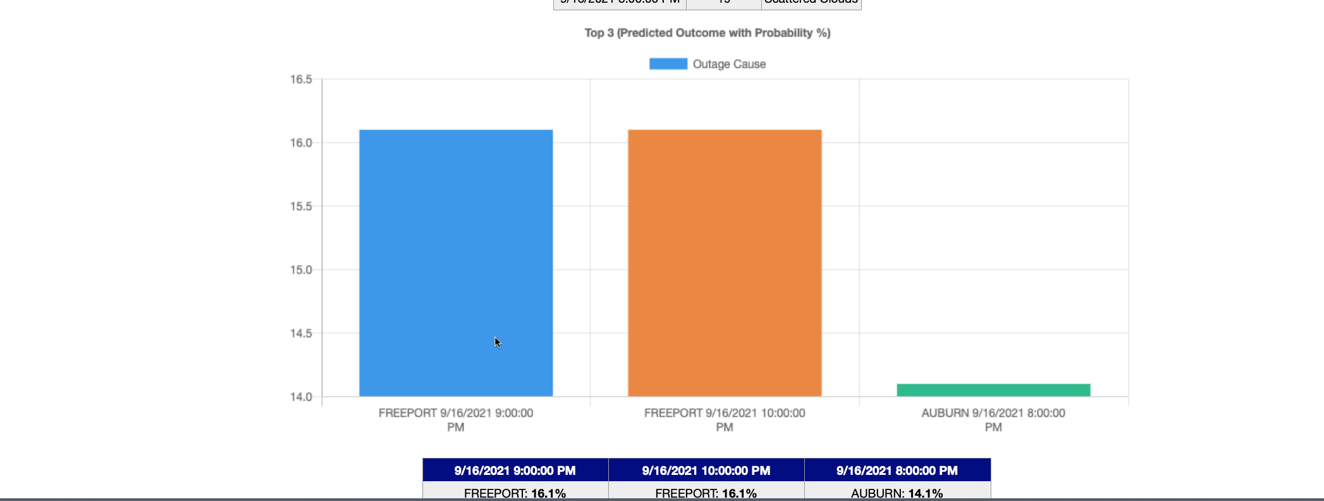
Why it’s important to learn how to improve after the storm?
Utilities can leverage technology to work smarter and not harder by proactively forecasting their risks. They can evaluate where outages will continue after the initial restoration.
More outages occur after the storm. Many communities are without heating or cooling for several days. Specifically, the risks continue for all communities because it is difficult to know which existing devices incurred damage. These devices may fail due to being hit by lighting or sustaining high wind damage. Companies that use their data can significantly reduce the time and cost of restoration.
What to do after the storm?
- Run outage reports and AI predictors to find storm and weather-related vulnerability
- Improve grid resiliency of feeders and substations by forecasting the highest probability of future outages.
- Implement new strategies to increase resiliency by adding solar, mobile battery units, micro-grids, and other DER.
- Evaluate lighting strikes and overlay sectional data to identify future device failures.
- Conduct fuse coordination study.
- Add the latest outage, AMI and SCADA data in PwrMetrix® and apply new AI models to train the AI predictors.
Why it’s cost-effective to use PwrMetrix®?
Few companies can afford data scientists to spend weeks manually managing the data.
These data scientists use tools, like Power BI or Tableau. However, these tools don’t provide decision material that can be easily acted upon. They cannot predict the time of failure, seasonality, and future survivability of existing devices. That’s because those tools don’t incorporate real-time weather, flood, or lighting data.
Large scale business intelligence companies require a full “rip and replace” of all systems to extract the data. These systems cost millions and are rarely updated in real-time.
Qualify Quality of Data
PwrMetrix® identifies issues regarding tracking outage quality data. These errors are easily remedied with an advanced detector.
Effective Use of Resources
Line crews can be managed during operational hours to decrease the power restoration risk and reduce labor costs. By using AI predictors built specifically for the utility industry, linemen and dispatchers can use their limited resources more efficiently.
Reduce Insurance Risk Costs
The insurance risk cannot be accurately assessed based on utilities existing outage data alone. The insurance assessor can see how the utility is using AI, data overlays and predictive maintenance to reduce the outage risks. The insurance assessor can insure future utility investments based on a proactive approach in mitigating risks to the communities.
Easy AI With Just A Click
The PwrMetrix® AI predictor continually ingests newly aggregated data to improve the predictor accuracy. PwrMetrix® AI predictors don’t require coding or the salaries of software developers. Overlaying different data points and training AI predictors built specifically for the utility industry, empower utilities to get better results, faster.
PwrMetrix® makes it easier to manage reliability and resiliency?
Utilities have massive amounts of data from various siloed systems (OMS, AMI, SCADA, EA, etc.). PwrMetrix® makes it easy to aggregate data from different systems to create reports and predict the future. Utilities can quickly improve reliability, increase resiliency, and manage their resources more efficiently with just a click of a button. By analyzing their data on demand, utilities can be more effective in weathering the storm.